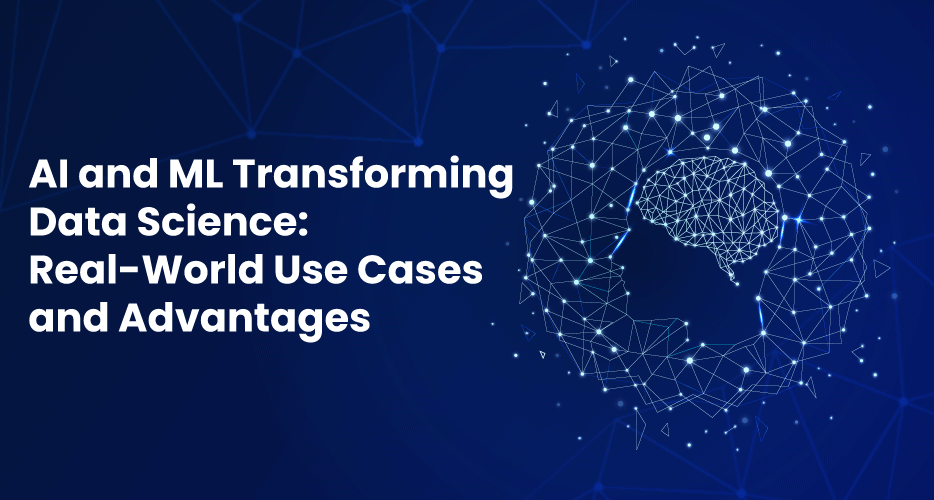
With the rise of AI and ML, organizations are more eager to utilize the potential of data fully. Harvard Business Review revealed shocking reviews about AI, according to the reports AI will bring $13 trillion into the global economy over the next decade. The stats show that AI is contributed a lot in offering substantial advantages to organizations. This contribution will drastically improve the organization’s profit margins and business operations.
Read the blog to learn how your organization can leverage AI to harness the full potential of data. In this blog, we will also talk about the use cases of AI/ML in data science and what benefits it offers to organizations.
Use Cases of AI/ML In Data
In today’s era, data is a vital resource for organizational growth and success. With the application of AI /ML, revolutionary results can be produced from data. Here is the list of AI ML use cases in the domain of data science.
1. Data Exploration and Insights:
AI/ML algorithms can be applied to explore and visualize complex data sets, uncovering hidden insights and correlations that may not be immediately apparent through traditional data analysis methods. Here is the list of processes AI can simplify in data exploration and getting useful insights.
- Data Visualization
- Data Summarization
- Automated Data Analysis
- Data Sampling and Sub-setting
- Data Clustering
- Data Classification
- Realtime Insights
2. Real-Time Data Processing:
AI/ML algorithms process and analyze data in real-time, enabling organizations to react swiftly to emerging trends, events, or anomalies. This capability is particularly valuable in industries where immediate insights drive critical decision processes. With the changing data and trends in the market, businesses have to keep updating their business policies to stay ahead of the competition.
Explore the critical processes where AI plays a pivotal role in delivering real-time data insights, helping organizations seize timely opportunities and make informed decisions.
- Realtime Dashboards
- Resource Allocation
- Streaming Data Processing
- Fraud Detection
- Anomaly Detection
3. Data Quality Improvement:
AI/ML models are widely used in organizations to detect and rectify data inconsistencies, anomalies, and missing values that can create disruption in the decision-making process. By improving data quality, and accuracy, organizations can make better-informed decisions based on accurate and reliable information. Here is a list of processes where AI plays a significant role in improving the data quality of an organization.
- Data Validation and Cleansing
- Data Governance
- Data Quality Assessment
- Duplicate Detection
- Data Profiling
4. Predictive Analytics:
Integrating AI/ML models with data engineering enables organizations to perform predictive analytics. These models can anticipate trends, identify patterns, and make forecasts, empowering businesses to take proactive actions and stay ahead of the competition. Below, you’ll find a compilation of processes where AI plays a pivotal role in predictive data analysis.”
- Pattern Recognition
- Regression Analysis
- Time Series Forecasting
- Risk Assessment
- Classification and Anomaly Detection
5. Personalization and Recommendation Systems:
AI/ML integration allows companies to create personalized experiences for individual customer behavior. By analyzing user behavior and preferences, recommendations can suggest products, services, or content that align with individual interests, enhancing customer satisfaction and engagement.
AI can enrich the personalization and recommendation systems of an organization by analyzing the data of individual users. Here’s how AI contributes to personalization and recommendation systems:
- User Profiling
- Content Filtering
- Realtime Personalization
- Cross-Platform Personalization
- Contextual Recommendation
6. Data Visualization and Reporting
AI algorithms convert raw data into visually appealing and constructive forms that help understand the hidden value of the data. Pie charts and histograms segregate information into useful and helpful formats that simplify the process of decision-making. Moreover, it highlights the important stats from the data that help generate hypotheses and in critical analysis of data. Here’s how AI simplifies the data visualization and reporting process of an organization.
- Automated Data Analysis
- Personalized Dashboards
- Predictive Analytics
- Anomaly Detection
- Automation of Reporting
- Realtime Reporting
- Data Security
- Data Structuring
7. Automated Mapping of Data
AI has been very helpful in the automated mapping of data. It leverages machine learning and advanced analytics to handle complex data integration challenges, making it a valuable tool in the field of data science. It recognizes data patterns and categorizes similar data points. Apart from this, AI also helps in various other aspects that help in the automated mapping of data. Here is the list of processes where AI integration plays an effective role in the automated mapping of data.
- Data Integration
- Pattern Recognition
- Semantic Analysis
- Data Transformation
- Data Cleansing
- Predictive Mapping
8. Data Pipeline Management
In data pipeline management, data quality assurance is very important for detecting any sort of anomalies in the data and AI can play a very significant role in improving data quality, detecting errors, and ensuring high quality of the content. Moreover, for seamless integration of data from different resources, AI smoothly performs data enrichment tasks. Furthermore, AI system sends you alerts of anomalies or unusual patterns in the data.
In short, AI handles all these issues effectively and eventually helps you manage data pipelines. Here are the processes given below where AI simplifies the process of data pipeline management.
- Data Quality Assurance
- Data Transformation and Enrichment
- Predictive Maintenance
- Performance Optimization
- Alerts and Anomaly Detection
- Resource Allocation
9. Automated Data Processing:
AI/ML algorithms can automate data extraction, transformation, and loading (ETL) processes, reducing manual intervention of employees and potential errors while processing large amounts of data. This automation speeds up data processing, making it more efficient and consistent. Here’s how AI ensures automated data processing.
- Data Transformation
- Data Enrichment
- Automation of Reporting
- Anomaly Detection
- Data Cleansing
- Pattern Recognition
Advantages:
- AI integration in data science helps identify patterns and popular trends in the market.
- AI quickly generates statistical insights to support decision-making.
- Perform Exploratory Data Analysis (EDA) for your business.
- Ensure high-speed mathematical processing.
- AI offers predictive analytics for better decision-making.
- Automation of intensive human tasks has helped the workforce to concentrate on other functions.
- AI/ML Increases efficiency and productivity in healthcare, insurance, pharma, marketing, and other industries.
- AI algorithms inform forecasting disasters and prepare for them beforehand.
- AI/ML integration in data leads to a reduction in human errors.
- Smart data-driven decision-making power leads to more fruitful results.
Final Thought
The AI ML integration in data science has unlocked a new era of data-driven decision-making which is offering potential benefits to organizations. Organizations can now fully harness the potential of data and improve revenue streams with better and more informed business policies. Moreover, AI is helping organizations unlock hidden insights, streamline operations, and gain a competitive edge.
AI/ML integration in data ensures validation and cleansing of data that helps organizations establish robust data governance, conduct data quality assessments, detect duplicates, and employ data profiling.
Moreover, the synergy between AI/ML and data science will continue to drive innovation, transforming industries, and opening new horizons for those ready to embrace the potential of data in its purest form. If you are looking to harness the full potential of data? Contact BITLogix today. The future is data-driven, and AI is leading the way.